Important Dates

Submission deadline
September 03, 2024
Acceptance Notification
October 04, 2024
Camera Ready
October 13, 2024
Registration
October 18, 2024
The International Conference on Complex Networks and their Applications aims at bringing together researchers from different scientific communities working on areas related to complex networks. Two types of contributions are welcome: theoretical developments arising from practical problems, and case studies where methodologies are applied. Both contributions are aimed at stimulating the interaction between theoreticians and practitioners.
Topics

Authors are encouraged to submit both theoretical and applied papers on their research in complex networks. Topics for the conference include, but are not limited to:
- Models of Complex Networks
- Structural Network Properties
- Complex Networks and Epidemics
- Community Structure and Discovery
- Networks, Smart Cities and Smart Grids
- Dynamics on and of Complex Networks
- Link Prediction
- Multiplex Networks
- Network Controllability
- Synchronization in Networks
- Algorithms for Network Analysis
- Complex Networks Mining
- Temporal and Spatial Networks
- Networks Visual Representation
- Large-scale Graph Analytics
- Social Reputation, Influence, and Trust
- Information Spreading in Social Media
- Rumor and Viral Marketing
- Financial and Economic Networks
- Complex Networks and Mobility
- Biological and Technological Networks
- Mobile Call Complex Networks
- Resilience and Robustness
- Graph Signal Processing
- Network Medicine
- Cognitive Network Science
- Networks for Physical Infrastructures
- Motif Discovery
- Political Networks
- Supply Chain Networks
- Complex Networks and Information Systems
- Recommendation Systems and Networks
- Complex Networks and CPS/IoT
- Network Neuroscience
- Quantifying Success through Social Network Analysis
- Bio-informatics and Earth Sciences Applications
Keynote Speakers

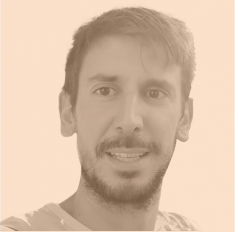
Federico BATTISTON
Central European University, Austria
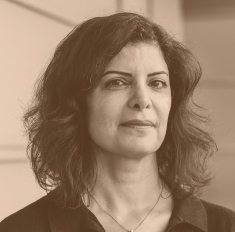
Tina ELIASSI-RAD
Northeastern University, USA

Frank EMMERT-STREIB
Tampere University, Finland
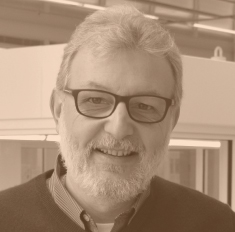
Filippo MENCZER
Indiana University, USA
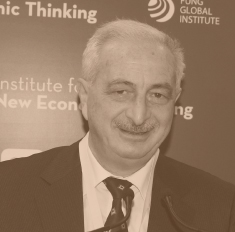
Luciano PIETRONERO
University of Rome “Sapienza”, Italy
Tutorials

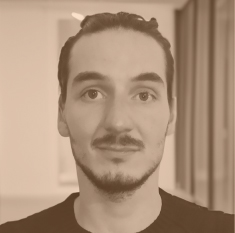
Alessandro GALEAZZI
University of Padova, Italy
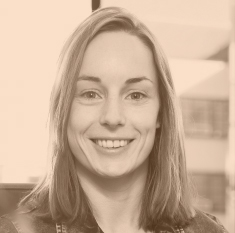
Clara STEGEHUIS
University of Twente, The Netherlands